The Future of Video Labeling: Revolutionizing Machine Learning with Innovative Tools
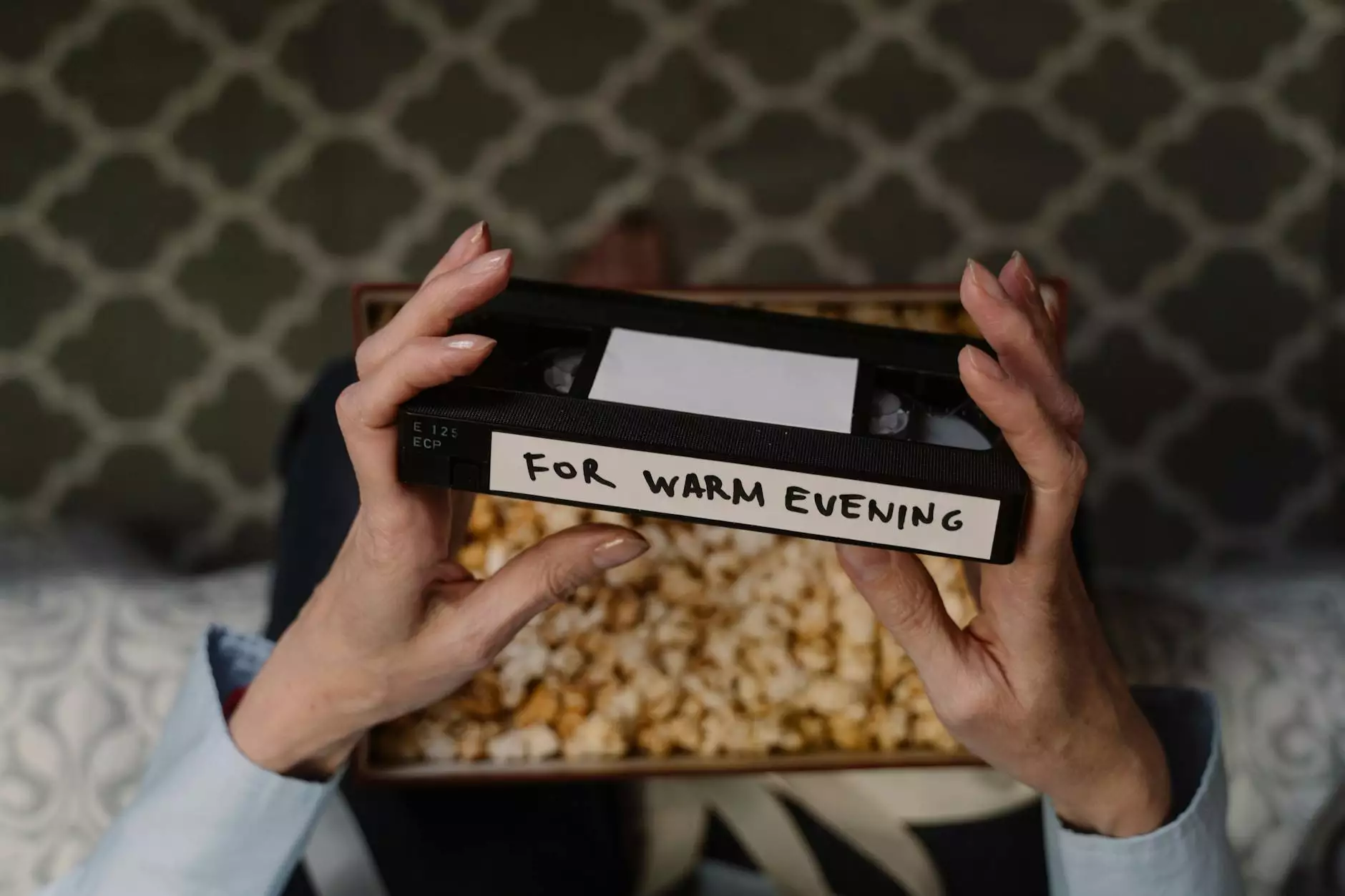
In the realm of machine learning, the need for accurate and efficient data annotation has never been more critical. One of the most exciting advancements in this sector is the development of specialized video labeling tool machine learning solutions. These tools are not just enhancing the way we annotate data but are also shaping the future of artificial intelligence.
Understanding Video Labeling Tools
Video labeling tools are software applications designed to facilitate the annotation of video data for various machine learning tasks. By providing a structured framework to label video content, these tools allow developers and data scientists to train more effective models. With the explosion of video content across digital platforms, the demand for reliable labeling solutions has skyrocketed.
The Importance of Data Annotation
Data annotation involves tagging or labeling data to make it understandable for machine learning algorithms. It provides the necessary training data that algorithms require to learn and make predictions. Without high-quality annotated data, machine learning models can fail to deliver accurate results, which is detrimental for businesses aiming to leverage AI technologies.
- Enhanced Model Accuracy: Well-annotated data leads to better predictive accuracy of machine learning models.
- Improved Training Efficiency: Automated annotation tools significantly reduce the time and effort required for data preparation.
- Scalability: Businesses can process large volumes of video content quickly and efficiently.
Key Features of Video Labeling Tools
The modern video labeling tool machine learning landscape is characterized by several innovative features that streamline the annotation process:
- Automated Annotation: Advanced algorithms can automatically detect and label objects within video frames, drastically reducing manual effort.
- User-Friendly Interface: Most video labeling tools come with intuitive interfaces, allowing users of all experience levels to annotate data with ease.
- Collaboration Features: Many platforms enable teams to work together in real-time, ensuring consistency and accuracy across annotations.
- Integration Capabilities: Seamless integration with other data handling tools and frameworks enhances productivity and workflow efficiency.
- Support for Multiple Formats: These tools frequently support a variety of video formats, making them adaptable to different use cases.
Applications of Video Labeling Tools in Machine Learning
Video labeling tools have broad applications across numerous industries with some notable examples including:
1. Autonomous Vehicles
In the automotive industry, accurate video labeling is crucial for the development of self-driving cars. By labeling objects such as pedestrians, road signs, and other vehicles in video data, machine learning models can better learn to navigate complex environments.
2. Security and Surveillance
Video surveillance systems utilize machine learning to detect unusual activities or threats. Annotated video data helps these systems improve their accuracy in identifying potential security breaches.
3. Healthcare
In medical settings, video labeling tools can assist in training algorithms for diagnosing conditions based on patient movement or behaviors captured in videos. For instance, monitoring patients for signs of distress can be enhanced through precise annotation.
4. Content Moderation
Social media platforms employ video labeling to identify and flag inappropriate content, ensuring a safer online environment. This requires sophisticated machine learning models trained on exhaustive datasets.
Benefits of Utilizing Video Labeling Tools
Integrating a reliable video labeling tool machine learning solution can lead to numerous advantages for businesses:
- Cost Efficiency: Reducing the time and labor involved in manual annotation leads to significant cost savings.
- Quality Control: Automated tools help maintain a consistent annotation process, minimizing human error.
- Faster Time-to-Market: Speeding up the data preparation phase allows businesses to expedite product development cycles.
- Adaptability: Many advanced tools are capable of performing complex tasks, allowing easy adaptation to various project requirements.
Choosing the Right Video Labeling Tool
With so many options on the market, selecting the appropriate tool for video labeling can be overwhelming. Here are key considerations to guide your decision-making process:
1. Project Requirements
Assess the specific needs of your project to determine what features are essential. For instance, do you need real-time collaboration or support for various video formats?
2. Ease of Use
A user-friendly interface can significantly affect the time it takes to get your team up to speed on the tool. Opt for solutions with a low learning curve.
3. Scalability
Your chosen tool should be able to grow with your organization. Ensure that it can handle increased data volumes as your needs evolve.
4. Support and Community
Reliable customer support and an active community can be invaluable when encountering challenges with software. Seek out tools with robust support channels.
Conclusion: A Paradigm Shift in Video Annotation
The emergence of effective video labeling tools is ushering in a new era in machine learning. By enabling faster, more accurate data annotation, these tools are not just facilitating the machine learning processes; they are fundamentally altering how we approach artificial intelligence.
As industries worldwide continue to harness the power of AI, investing in advanced video labeling tool machine learning solutions will be essential for maintaining a competitive edge. Businesses like KeyLabs.ai are at the forefront of this revolution, providing innovative platforms that meet the ever-growing demands of data annotation.
Embrace the future of AI today by leveraging the capabilities of video labeling tools. The path to robust, intelligent systems starts with precise and thorough data annotation.